The paper introduces AlphaGo Zero, the latest evolution of [AlphaGo](https://deepmind.com/research/alphago/), the first computer program to defeat a world champion at the ancient Chinese game of Go. Zero is even more powerful and is arguably the strongest Go player in history.
Previous versions of AlphaGo initially trained on thousands of human amateur and professional games to learn how to play Go. AlphaGo Zero skips this step and learns to play simply by playing games against itself, starting from completely random play. In doing so, it quickly surpassed human level of play and defeated the [previously published](http://www.nature.com/nature/journal/v529/n7587/full/nature16961.html?foxtrotcallback=true) champion-defeating version of AlphaGo by 100 games to 0.
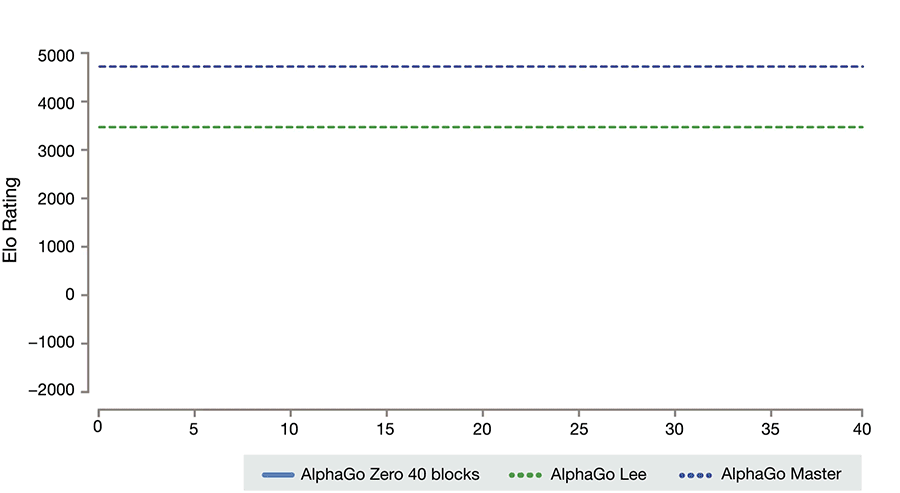
It is able to do this by using a novel form of [reinforcement learning](https://en.wikipedia.org/wiki/Reinforcement_learning), in which AlphaGo Zero becomes its own teacher. The system starts off with a neural network that knows nothing about the game of Go. It then plays games against itself, by combining this neural network with a powerful search algorithm. As it plays, the neural network is tuned and updated to predict moves, as well as the eventual winner of the games.
This updated neural network is then recombined with the search algorithm to create a new, stronger version of AlphaGo Zero, and the process begins again. In each iteration, the performance of the system improves by a small amount, and the quality of the self-play games increases, leading to more and more accurate neural networks and ever stronger versions of AlphaGo Zero.
This technique is more powerful than previous versions of AlphaGo because it is no longer constrained by the limits of human knowledge. Instead, it is able to learn tabula rasa from the strongest player in the world: AlphaGo itself.
It also differs from previous versions in other notable ways.
它还有其他一些有别于之前版本的显著的区别:
- AlphaGo Zero only uses the black and white stones from the Go board as its input, whereas previous versions of AlphaGo included a small number of hand-engineered features.
-It uses one neural network rather than two. Earlier versions of AlphaGo used a “policy network” to select the next move to play and a ”value network” to predict the winner of the game from each position. These are combined in AlphaGo Zero, allowing it to be trained and evaluated more efficiently.
- AlphaGo Zero does not use “rollouts” - fast, random games used by other Go programs to predict which player will win from the current board position. Instead, it relies on its high quality neural networks to evaluate positions.
All of these differences help improve the performance of the system and make it more general. But it is the algorithmic change that makes the system much more powerful and efficient.